Changing Narratives: How Inclusive Quality Data Can Reduce Inequalities for Black Women
Written by Ester Pinheiro, Communications Officer – Spanish, Equal Measures 2030 in conversation with the CEOs of Data for Black Lives, Yeshimabeit Milner and DataedX, Brandeis Marshal
The under- and mis-representation of Black women in data is an alarming issue. Data stigmatization coupled with the scarcity of data representation erases the perspectives and experiences of Black women and risks perpetuating and exacerbating existing inequalities, biases, and prejudices.
According to a paper on public perceptions of Black Women by Northwestern University and IPR, Black girls in the US suffer from “adultification”. They are seen as more dangerous, and sexually aware, thus influencing perceptions of them as deserving of harsher punishments than their peers. As the paper states, “these findings have important implications for understanding the general public’s potential role in shaping the punitive experiences of Black girls and raise questions about the consequences of their punishment for democracy.”
Yeshimabeit Milner, CEO of Data for Black Lives, underscores how Black women are disproportionately represented, particularly in criminal justice data, due to historical misrepresentations – “We’ve been bombarded with historically negative portrayals and stereotypes of Black women. Those media narratives and agendas were shaped by bad or fake data and only drive further policies that make Black women even more vulnerable.”
One of the most prevailing myths Black women suffer from is the crack baby myth. Milner points out that “in the 80s, there was this idea that there’s all these black women on crack, and they’re giving birth to these babies who are going to be a threat to society. However, when you look back 20–30 years later, those babies were the ones going to college. A ‘bad child’s outcome’ didn’t depend on whether their mother was on crack, it was down to poverty, to lack of access to resources, such as education, or healthcare.”
Data is key for demystifying racial biases
When it comes to the tech industry and its products, Black women and other minorities are under-represented. To buck these trends and increase representation we need effective policies. We need policies that are driven by and have their impact measured using quality data that includes race and gender critical perspectives.
“Using data about the state, use and inequities thrust upon historically excluded communities, like Black people, provides a historical, cultural and political context that informs where existing policies lack. Data can either be used to help us remedy these intentional oversights of our past or expand the oppression. Data is a marker on whether we are progressing or regressing in our tech policy development” says Brandeis Marshall, the Founder and CEO of DataedX’s Group.
DataedX’s collaborative efforts have revealed racial and gender disparities in data fields, providing quantitative evidence for qualitative insights. Conscious of these disparities, the group produced several recommendations to ensure that data fields did not leave minorities behind, and these were reflected in the Blueprint for an AI Bill of Rights released by the White House in October 2022.
Similarly, Data for Black Lives sees data as a tool to denounce and to demand accountability. As Milner puts it, “data is collective action to partly have counter-narratives as well as reverse engineer decades of policies, programs and unwritten rules Black women follow of those who are ruling society and power scene.”
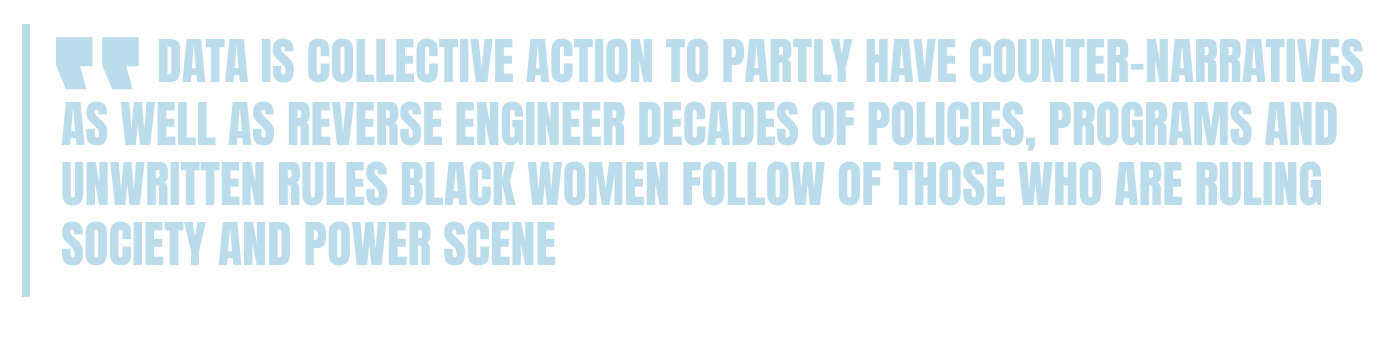
It is not just about disaggregated data: racist algorithms
Whilst diverse and representative disaggregated data is vital in avoiding the perpetuation of biases, we must also pay attention to algorithms and their power in decision-making. As the CEO of Data for Black Lives points out, “it’s about what and how are these patterns, how are the data systems of today categorizing. First, how are algorithms recognizing and able to detect someone’s race and gender? And once they have that information, how are algorithms categorizing based on, once again, historic patterns of discrimination?”
Milner gives an example she has shared in the White House and in the US Congress regarding artificial intelligence and civil rights. She hopes to dethrone the FICO model in-credit mark as the predominant indicator of risk in terms of lending. “It’s the most powerful algorithm in our country because over 90% of the population is scored by it before renting”.
“A lot of us are told, especially Black women, ‘the reason you have a low credit score is because of missed payments or because of this or of all these factors (…)’, but we can never tell what the real factors are, because this is a proprietary algorithm owned by a private company.”
Among Black mothers in the US, more than 4 out of 5 (3.0 million of 3.7 million women, or 81.1%) are breadwinners and are the ones tasked with securing the next generation of their families. “A lot of us are tasked with taking care of our entire extended families, and we should have the right to be able to have credit and not have to pay more for the same products: whether it’s car insurance, home loans, Medical Care – it really makes a difference.”
On the other hand, according to some algorithms like the FICO scoring system, because they are Black and because they are women, they are automatically deemed as less worthy. “Even though it’s illegal in the US to deny someone housing based on race gender, you can’t sue an algorithm, so this is what a lot of us have to face as Black women.”
For Milner, this is why disaggregated data is important; to get under the hood of some of these very powerful algorithms and understand how they are categorizing and detecting someone’s race and gender.
Looking forward, Brandeis Marshal reveals one hope she has for the next few years regarding data governance and advocacy. “In the future, data, and AI governance legislation will be created, likely with pressure from the people, that the state and federal governments will slightly expand, codify in law and develop enforcement protocols. The focus has been on regulating Big Tech in recent years, but I think the progressive data and AI governance we’ll see will originate from small businesses first.”